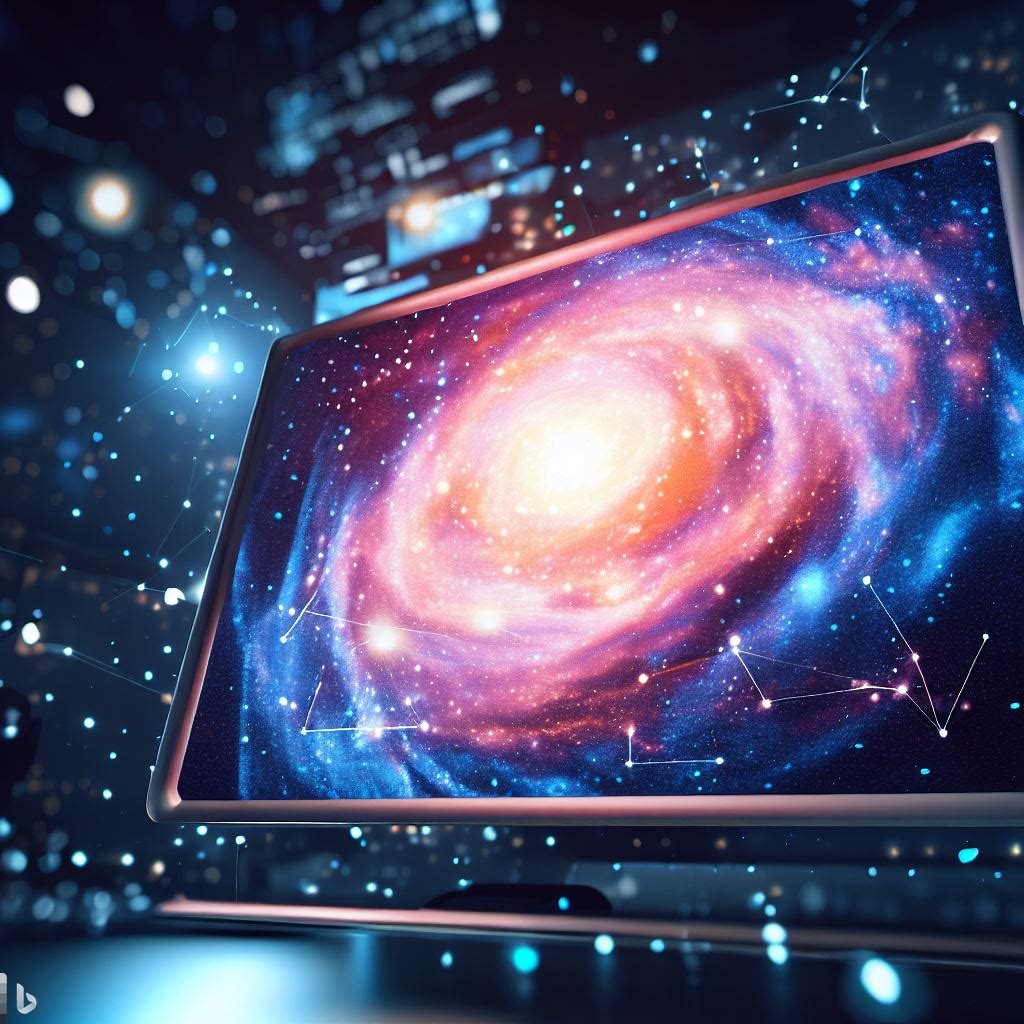
This Ph.D. thesis falls into the exciting field of Astroinformatics by employing advanced machine learning techniques to detect Active Galactic Nuclei (AGN) through the analysis of optical variability in light curves coming from the VLT Survey Telescope (VST) and Zwicky Transient Facility (ZTF) with the aim of building tools that can be applied to the data coming from Rubin Observatory’s Legacy Survey of Space and Time (LSST).
AGN are enigmatic and highly energetic celestial objects harboring supermassive black holes at their cores. Unveiling the presence of AGN within galaxies is crucial for understanding their formation, evolution, and the intricate relationship between supermassive black holes and their host galaxies. Due to their variability they are hard to be detected by considering single exposures in optical bands.
The research proposed here revolves around the application of cutting-edge machine learning algorithms to automate the identification and classification of AGN based on the optical variability observed in their light curves. These, representing the temporal evolution of a celestial object’s brightness, offer valuable insights into the dynamic processes occurring in AGN. This thesis will exploit the potential of machine learning in dealing with such complex astronomical datasets to uncover AGN efficiently.
The primary objectives of this study include:
- Data Collection and Preprocessing: Gathering optical light curve data from a variety of astronomical surveys and telescopes. Implementing preprocessing steps to clean and standardize the data for analysis.
- Feature Engineering: Extracting informative features from the light curves, such as time-domain statistical measures, periodicity indicators, and spectral characteristics, to capture AGN-specific variability patterns.
- Machine Learning Models: Developing and fine-tuning of supervised machine learning models, including deep neural networks, support vector machines, and random forests, to classify AGN based on the extracted features.
- Model Evaluation and Validation: Assessing the performance of the machine learning models through rigorous cross-validation and benchmarking against established AGN catalogs. Evaluating the robustness of the models in distinguishing AGN from other astronomical sources.
- Interpretability and Generalization: Investigating the interpretability of the models to gain insights into the physical processes driving AGN variability. Characterizing the non-confirmed AGN in order to identify potentially interesting sources for follow-up studies. Ensuring the generalizability of the developed algorithms across different datasets and observational conditions.
The outcome of this research has the potential to strongly improve AGN detection by offering a powerful and automated approach to identify these intriguing objects in large-scale optical surveys. This work will then contribute to our broader understanding of AGN and their role in shaping the cosmos while showcasing the broader applicability of machine learning methods in astrophysical research. Ultimately, it paves the way for deeper insights into the mysteries of the universe’s most energetic phenomena.
This work is part of a framework of studies already started by the local community both at the Observatory and at the Physics Department, see for example: Cavuoti+14; De Cicco+15,19,21,22; D’Isanto+16.
Riferimenti: Stefano Cavuoti & Demetra De Cicco.